September 5, 2024
Navigating the Autonomous AI Revolution in the Enterprise
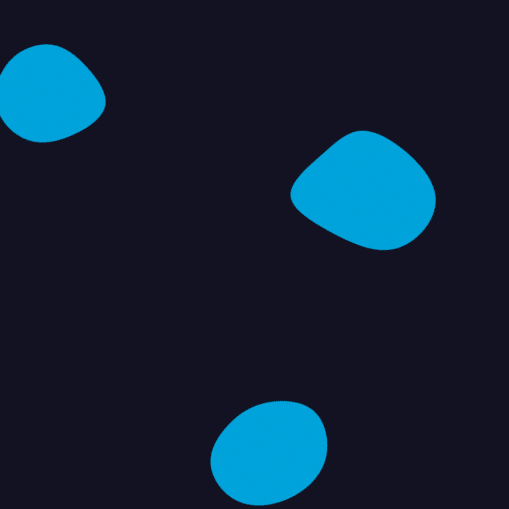
As artificial intelligence continues to evolve at a breakneck pace, many organizations are grappling with how to effectively implement and manage AI systems at scale. A new guidance paper titled Autonomous AI in the Enterprise: A Fictional Case Study by John Willis, Tapabrata Pal, Ben Grinnell, John Rauser, Damon Edwards, and Joseph Enochs offers valuable insights for technology leaders looking to chart a course through this complex landscape.
The paper presents a fictional scenario at a company called Data Unlimited, where executives are debating the merits and risks of aggressively adopting autonomous AI technology. Through their discussions and planning, several key themes emerge that are highly relevant for any enterprise embarking on an AI transformation journey.
Balancing Opportunity and Risk
The paper highlights the tension between AI’s immense potential to drive innovation and efficiency and the very real risks it poses if not implemented thoughtfully. The CEO, Anna Lee, paints an exciting vision of AI revolutionizing their business and catapulting them ahead of the competition. However, the CIO, Tom Harding, urges caution based on his experience with previous technology shifts that promised easy wins but led to unintended consequences.
This push-pull between opportunity and risk is a central challenge for technology leaders. The paper suggests that success lies in finding a middle ground—moving quickly enough to capture AI’s benefits while also putting guardrails in place to manage downside risks.
The Specter of Shadow AI
One of the biggest risks highlighted is the emergence of “shadow AI”—AI systems and tools adopted by individual business units without proper oversight or integration with enterprise architecture. The ease of deploying modern AI solutions makes this a particularly acute challenge.
To combat shadow AI, the paper recommends:
- Establishing a centralized AI governance committee
- Implementing a review process for all AI projects
- Creating a secure, scalable infrastructure for AI experimentation and production
- Setting clear guidelines for data management and model development
By providing a governed but flexible environment for AI adoption, enterprises can enable innovation while maintaining control.
Managing Technical Debt in AI Systems
The paper draws on insights from Google’s seminal work on technical debt in machine learning systems to highlight how AI can accumulate “hidden” technical debt in ways traditional software does not. This includes issues like unstable data dependencies, correction cascades, and configuration sprawl.
To manage this unique form of technical debt, the authors recommend:
- Regular debt assessments using specialized tools
- A culture of continuous refactoring
- Modular, reusable AI components
- Robust documentation and reproducibility practices
By proactively addressing technical debt, organizations can build AI systems that remain maintainable and scalable over time.
Cross-Functional Collaboration is Key
A recurring theme throughout the paper is the critical importance of cross-functional collaboration in successful AI adoption. The fictional case study shows how partnerships between IT, security, data science, and business units are essential for developing a cohesive AI strategy.
The paper suggests creating a cross-functional AI governance committee to oversee the AI road map and make strategic decisions. It also emphasizes the need for ongoing communication and knowledge sharing across departments involved in AI development.
Security and Compliance Considerations
As AI systems often deal with sensitive data and can introduce novel vulnerabilities, security is a major focus of the paper. The CISO character, Sarah Jung, raises important points about emerging AI security risks, including prompt injection attacks, training data poisoning, and model theft.
The paper recommends:
- Incorporating security considerations from the outset of AI projects
- Educating employees about AI-specific security risks
- Implementing robust access controls and data governance
- Regular security audits of AI systems
By prioritizing security, enterprises can mitigate risks and build trust in their AI initiatives.
Building a Foundation for the Future
While the paper’s title refers to “autonomous AI,” the authors are careful to note that true autonomy in AI systems is still largely aspirational. Instead, they argue that enterprises should focus on building a solid foundation for generative AI in the near term, which will position them well for more advanced AI capabilities in the future.
Key elements of this foundation include:
- A centralized, secure, and scalable AI infrastructure
- Clear governance processes and guidelines
- Best practices for data management and model development
- A culture of responsible AI adoption
By getting these fundamentals right, organizations can create an environment where AI can thrive while remaining aligned with business objectives and ethical considerations.
Conclusion
Autonomous AI in the Enterprise offers a thought-provoking and practical guide for technology leaders navigating the complexities of large-scale AI adoption. By presenting insights through a relatable fictional scenario, the authors make abstract concepts concrete and actionable.
This paper is a must-read for any technology leader grappling with AI strategy, governance, or implementation challenges. It offers a balanced perspective on AI’s transformative potential and the very real challenges it presents.
To dive deeper into these concepts and gain additional insights, we encourage you to download the full paper for free. Armed with the knowledge and strategies outlined in this guidance, you’ll be better equipped to lead your organization successfully through the AI revolution.
Leave a Comment
More Like This
How I Built a Google Docs Add-On in Three Hours (Using Vibe Engineering)
So, I'm writing a book with Steve Yegge (famous for his 20 years at…
How I Broke My 20-Year SPSS Habit To Vibe Code Something in a Python Colab Notebook
So, I'm writing a book with Steve Yegge (famous for his 20 years at…
Flow Engineering Immersion Course: Transform How Teams Work Together
We're excited to announce that the Flow Engineering Immersion Course, the third installment in…
Becoming a Better Leader Part 2: Building Trust Through Understanding
Trust is the foundation of effective leadership. Yet in many organizations, trust remains elusive—especially…
No comments found